nnDetection: A Self-configuring Method for Medical Object Detection
Published in Medical Image Computing and Computer Assisted Intervention–MICCAI 2021, 2021
Recommended citation: Baumgartner, Michael and Jaeger Paul, et al. "nnDetection: a self-configuring method for medical object detection." Medical Image Computing and Computer Assisted Intervention–MICCAI 2021: 24th International Conference, Strasbourg, France, September 27–October 1, 2021, Proceedings, Part V 24. Springer International Publishing, 2021. https://link.springer.com/chapter/10.1007/978-3-030-87240-3_51
Simultaneous localisation and categorization of objects in medical images, also referred to as medical object detection, is of high clinical relevance because diagnostic decisions often depend on rating of objects rather than e.g. pixels. For this task, the cumbersome and iterative process of method configuration constitutes a major research bottleneck. Recently, nnU-Net has tackled this challenge for the task of image segmentation with great success. Following nnU-Net’s agenda, in this work we systematize and automate the configuration process for medical object detection. The resulting self-configuring method, nnDetection, adapts itself without any manual intervention to arbitrary medical detection problems while achieving results en par with or superior to the state-of-the-art. We demonstrate the effectiveness of nnDetection on two public benchmarks, ADAM and LUNA16, and propose 11 further medical object detection tasks on public data sets for comprehensive method evaluation. Code is at this URL.
Abstract was presented as an oral presentation during BVM 2022 and was awarded the best presentation award.
Our submission to the MELA 2022 was based on nnDetection and ranked 3rd place. Three out of five top performing solutions, including the winning solution, were based on nnDetection.
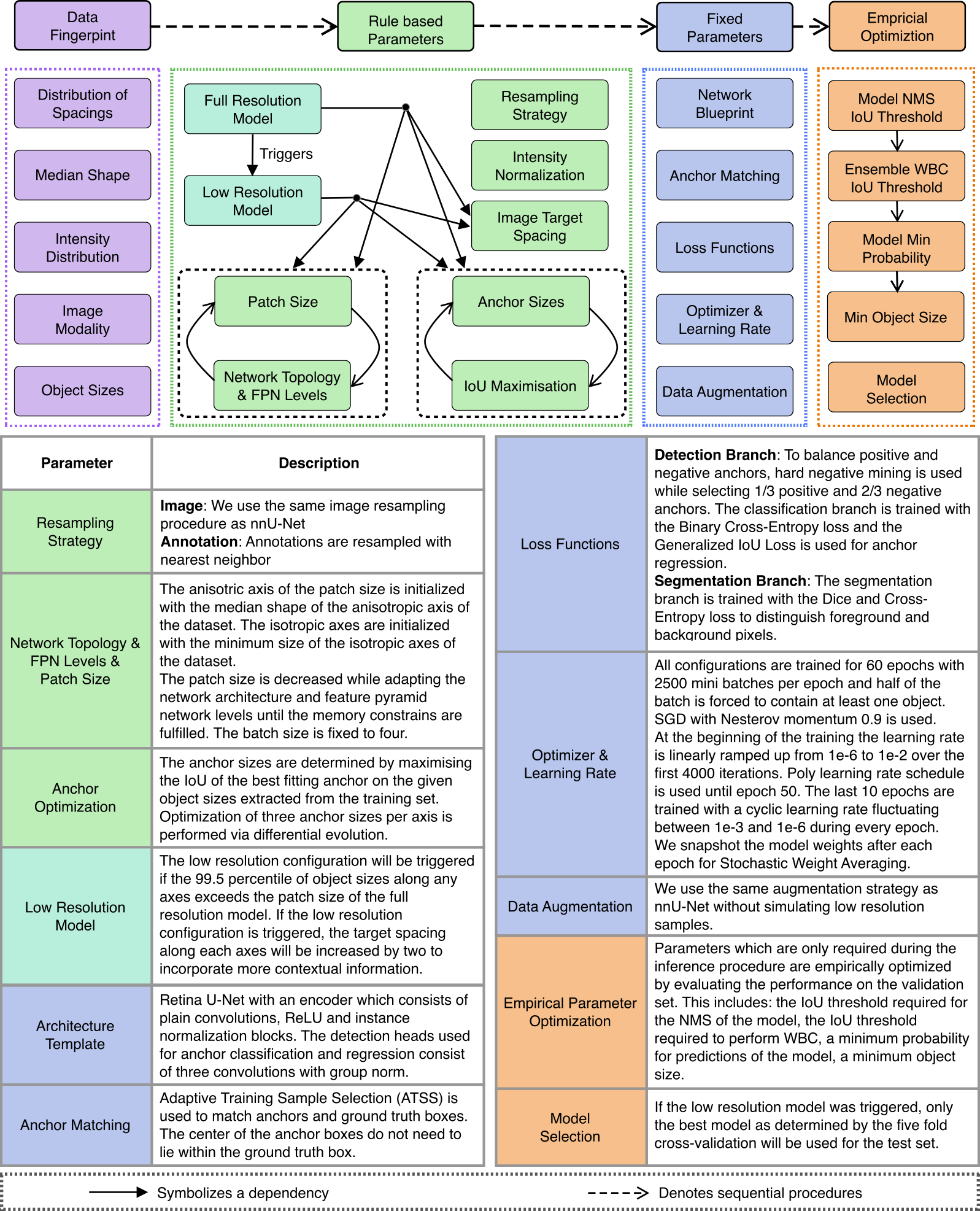